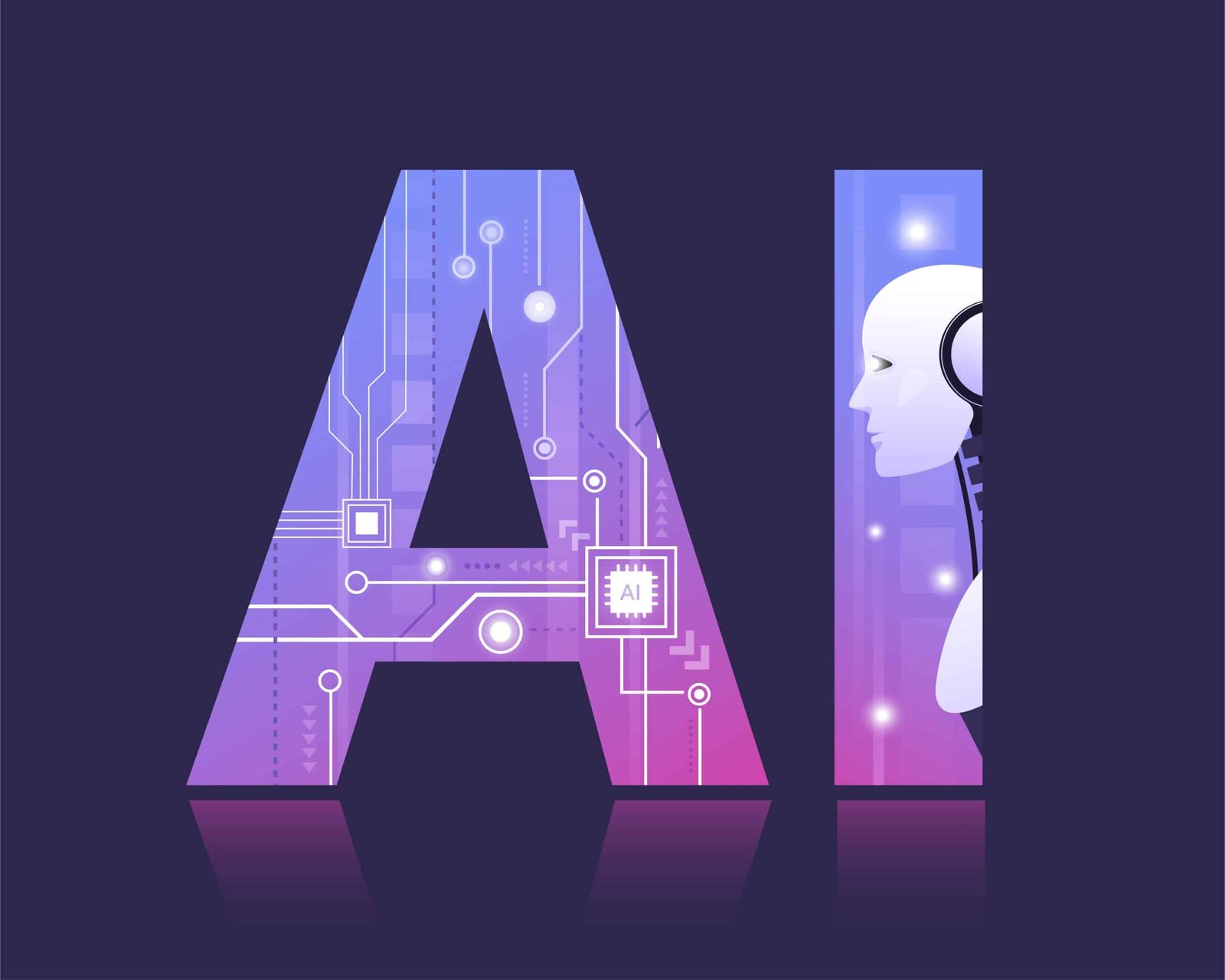
In today’s data-driven landscape, the convergence of Generative AI and data analytics has ushered in a new era of business intelligence. Traditional data analytics primarily focuses on interpreting historical data to identify patterns and trends. In contrast, Generative AI not only analyzes existing data but also creates new possibilities by filling in gaps, generating predictions, and formulating entirely new datasets to address complex business challenges.
The exponential growth of data necessitates advanced analytical tools. According to recent reports, 94% of data leaders believe AI enhances data focus and management, highlighting the critical role of AI in modern data analytics. This surge in data generation underscores the need for sophisticated tools like Generative AI to process and derive actionable insights efficiently.
Businesses across various sectors are harnessing Generative AI-powered analytics to transform operations and gain a competitive edge. From fraud detection and personalized marketing to predictive maintenance and demand forecasting, the applications are vast and impactful. For instance, financial institutions have reported a 45% increase in fraud detection rates after implementing Generative AI systems, significantly reducing false positives.
In the retail sector, companies utilizing Generative AI for demand forecasting have achieved unprecedented accuracy, optimizing inventory management and reducing excess stock. By analyzing complex market trends and consumer behavior, AI-driven analytics enable businesses to anticipate demand fluctuations with greater precision, leading to reduced operational costs and higher profitability. Now, let’s explore some real-world Generative AI use cases by industry.
1. Fraud DetePicture 1.jpg11ction & Cybersecurity – How PayPal Reduced Fraudulent Transactions
The Challenge
Online transactions are a prime target for fraudsters. PayPal, handling billions of transactions daily, needed a better fraud detection system that could keep up with evolving cyber threats and detect fraudulent activities before they caused financial losses.
How Generative AI Solved It
PayPal implemented Generative AI-powered fraud detection that:
- Generated synthetic fraud cases to train its machine learning models
- Simulated new fraud patterns that traditional systems might miss
- Analyzed user behavior in real-time to detect suspicious transactions
Results
After deploying AI-driven fraud detection, PayPal reduced fraudulent transactions by 40% while maintaining a seamless user experience for legitimate customers.
Takeaway: If your business involves digital transactions, Generative AI can identify fraud before it happens, saving you millions in losses.
2. Personalized Customer Experience – How Netflix Keeps Users Hooked
The Challenge
Netflix wanted to improve user engagement and retention by offering hyper-personalized recommendations. Traditional recommendation engines analyzed past viewing history, but they lacked deep personalization based on emotions, visuals, and detailed content preferences.
How Generative AI Solved It
Netflix used Generative AI-powered analytics to:
- Generate personalized thumbnails based on user preferences (e.g., if you love comedies, the thumbnail will show a funny scene)
- Predict what users want to watch before they search
- Create AI-driven trailers and previews that are tailored to different audience segments
Results
This AI-driven personalization increased user engagement by 35%, leading to longer watch times and lower subscription cancellations.
Takeaway: Whether you run an e-commerce store, an entertainment platform, or a content website, Generative AI can personalize user experiences to boost customer retention.
3. Predictive Maintenance – How Rolls-Royce Prevents Aircraft Failures
The Challenge
Aircraft engine failures are not just costly, they can be life-threatening. Rolls-Royce needed a way to predict engine failures before they occurred, preventing unexpected maintenance costs and flight delays.
How Generative AI Solved It
Rolls-Royce implemented AI-driven predictive maintenance, which:
- Analyzed live engine data from sensors to detect early warning signs
- Generated simulated failure scenarios to train AI models
- Predicted potential malfunctions before they happened
Results
By leveraging AI-powered data analytics, Rolls-Royce reduced engine failures by 30%, improving flight safety and operational efficiency.
Takeaway: Businesses in manufacturing, transportation, or logistics can use Generative AI to predict and prevent costly equipment failures.
4. Supply Chain Optimization – How Amazon Predicts Demand
The Challenge
Amazon handles millions of shipments daily, and any supply chain disruption can lead to huge losses. The company needed a way to predict demand more accurately and optimize inventory levels before shortages or excess stock occurred.
How Generative AI Solved It
Amazon’s AI-powered analytics:
- Simulated demand trends based on external factors like weather, holidays, and economic conditions
- Generated supply chain models to improve warehouse efficiency
- Predicted customer buying behavior weeks in advance
Results
With Generative AI, Amazon reduced inventory waste by 20% and improved delivery efficiency, keeping products in stock without overloading warehouses.
Takeaway: Whether you own a small retail store or a global supply chain, AI-powered analytics can predict demand more accurately and reduce waste.
5. Financial Forecasting – How JPMorgan Chase Improves Investment Strategies
The Challenge
Investment firms rely on accurate financial predictions, but traditional models often fail during unexpected market shifts. JPMorgan Chase needed a way to simulate various financial scenarios to guide investment decisions.
How Generative AI Solved It
JPMorgan Chase used AI-driven financial modeling, which:
- Generated simulated stock market trends based on real-time data
- Predicted financial risks before they impacted portfolios
- Created alternative investment strategies for various economic conditions
Results
This AI-driven approach reduced investment risks and improved returns by 25%, allowing JPMorgan Chase to stay ahead of market fluctuations.
Takeaway: If your business involves investments, loans, or risk management, Generative AI can help you predict financial outcomes with greater accuracy.
6. Healthcare Diagnostics – How Mayo Clinic Improves Disease Detection
The Challenge
Doctors rely on medical imaging and patient records to diagnose diseases, but sometimes critical details are missed, leading to delayed treatment. Mayo Clinic needed an AI-driven system to enhance diagnostics and assist doctors.
How Generative AI Solved It
Mayo Clinic deployed AI-driven medical analytics, which:
- Generated enhanced medical images to detect abnormalities earlier
- Analyzed patient history to predict disease risks
- Suggested personalized treatment plans for better outcomes
Results
AI-powered diagnostics improved early disease detection rates by 40%, allowing doctors to start treatments sooner and save more lives.
Takeaway: AI-powered data analytics can revolutionize healthcare, insurance, and patient care services.
Final Thoughts: Why Generative AI in Data Analytics Matters
Generative AI is not just a fancy tech innovation, it’s reshaping industries by solving real-world problems. From fraud detection to predictive maintenance, AI-driven analytics is helping companies reduce risks, optimize operations, and improve customer experiences.
Whether you’re an entrepreneur, a data analyst, or a business owner, understanding Generative AI use cases can give you an edge in an AI-driven future. Are you ready to harness the power of Generative AI for your business?