Quantum machine learning (QML) represents a fascinating intersection of two of the most transformative fields in contemporary technology: quantum computing and machine learning. As the demand for more sophisticated data analysis techniques grows, traditional machine learning methods often struggle with the sheer volume and complexity of data. Quantum computing, with its unique principles of superposition and entanglement, offers a promising alternative that could revolutionise how we process information.
By harnessing the power of quantum mechanics, QML aims to enhance machine learning algorithms, potentially leading to breakthroughs in various domains, from healthcare to finance. The concept of QML is not merely an academic curiosity; it is a burgeoning field that has garnered significant attention from researchers and industry leaders alike. The integration of quantum computing into machine learning frameworks could enable the development of algorithms that are exponentially faster than their classical counterparts.
This potential for speed and efficiency is particularly appealing in an era where data is generated at an unprecedented rate. As we delve deeper into the intricacies of quantum machine learning, it becomes essential to understand the foundational principles of quantum computing that underpin this innovative approach.
Summary
- Quantum machine learning combines the principles of quantum mechanics and machine learning to solve complex problems.
- Quantum computing uses quantum bits or qubits to process and store information, allowing for parallel processing and increased computational power.
- Quantum machine learning has the potential to revolutionize industries such as finance, healthcare, and cybersecurity by solving complex optimization and pattern recognition problems.
- Challenges in quantum machine learning include the need for error correction, limited qubit connectivity, and the high cost of building and maintaining quantum computers.
- Quantum machine learning algorithms such as quantum support vector machines and quantum neural networks have the potential to outperform classical machine learning algorithms in certain tasks.
Understanding Quantum Computing
The Power of Qubits
Classical computers use bits as the smallest unit of data, which can exist in one of two states: 0 or 1. In contrast, quantum computers utilise quantum bits, or qubits, which can exist in multiple states simultaneously due to the phenomenon known as superposition.
Unleashing Computational Power
This ability allows quantum computers to perform many calculations at once, significantly increasing their computational power. Entanglement is another critical principle of quantum mechanics that plays a vital role in quantum computing. When qubits become entangled, the state of one qubit becomes dependent on the state of another, regardless of the distance separating them.
Enhancing Machine Learning
This interconnectedness enables quantum computers to solve complex problems more efficiently than classical systems. For instance, while a classical computer might take an impractically long time to factor large numbers—a task crucial for cryptography—a quantum computer could potentially accomplish this in a fraction of the time. Understanding these principles is essential for grasping how quantum machine learning can exploit the advantages of quantum computing to enhance machine learning processes.
The Potential of Quantum Machine Learning
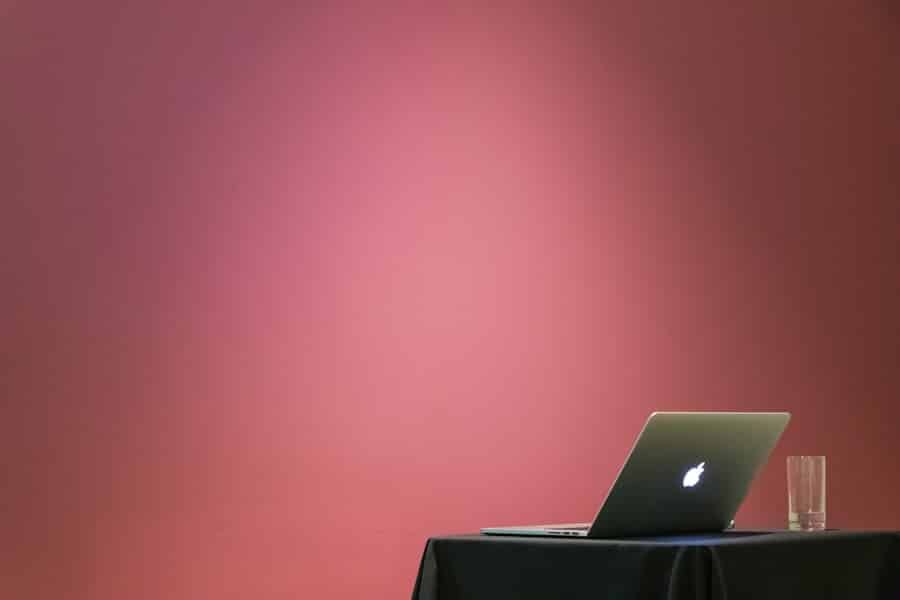
The potential applications of quantum machine learning are vast and varied, spanning numerous fields and industries. One of the most promising areas is in optimisation problems, where QML could significantly outperform classical algorithms. For example, in logistics and supply chain management, companies often face complex optimisation challenges that involve numerous variables and constraints.
Quantum algorithms could provide solutions that are not only faster but also more accurate, leading to substantial cost savings and efficiency improvements. Moreover, QML holds promise in the realm of drug discovery and personalised medicine. Traditional machine learning techniques can analyse vast datasets to identify potential drug candidates or predict patient responses to treatments.
However, these methods can be limited by their reliance on classical computational power. Quantum machine learning could enable researchers to model molecular interactions at a level of detail previously unattainable, accelerating the discovery of new therapies and improving patient outcomes. The ability to process and analyse complex biological data more effectively could lead to significant advancements in healthcare.
Challenges and Limitations of Quantum Machine Learning
Despite its immense potential, quantum machine learning faces several challenges and limitations that must be addressed before it can be fully realised. One significant hurdle is the current state of quantum hardware. While there have been remarkable advancements in quantum computing technology, many existing quantum systems are still prone to errors due to decoherence and noise.
These issues can lead to inaccurate results when running QML algorithms, necessitating the development of error-correcting codes and more robust quantum architectures. Another challenge lies in the complexity of designing effective QML algorithms. While some theoretical frameworks exist, translating these concepts into practical applications requires a deep understanding of both quantum mechanics and machine learning principles.
Researchers must navigate a steep learning curve as they work to create algorithms that can leverage quantum advantages while remaining accessible to practitioners in the field. Additionally, there is a pressing need for interdisciplinary collaboration between quantum physicists and machine learning experts to bridge the gap between theory and application.
Quantum Machine Learning Algorithms
The landscape of quantum machine learning algorithms is diverse, with various approaches being explored to harness the power of quantum computing for machine learning tasks. One notable example is the Quantum Support Vector Machine (QSVM), which adapts the classical support vector machine algorithm for use on quantum computers. By leveraging quantum superposition, QSVM can potentially classify data points more efficiently than its classical counterpart, particularly in high-dimensional spaces.
Another promising algorithm is the Quantum Principal Component Analysis (QPCA), which aims to identify the principal components of a dataset using quantum techniques. This approach could significantly reduce the time required for dimensionality reduction, a common preprocessing step in many machine learning workflows. Additionally, Variational Quantum Eigensolver (VQE) techniques are being explored for optimisation problems within QML frameworks, allowing for hybrid approaches that combine classical and quantum resources effectively.
As research progresses, new algorithms are continually being developed that seek to exploit unique quantum properties for specific machine learning tasks. The ongoing exploration of these algorithms will be crucial in determining how effectively QML can be integrated into existing machine learning paradigms.
Real-world Applications of Quantum Machine Learning
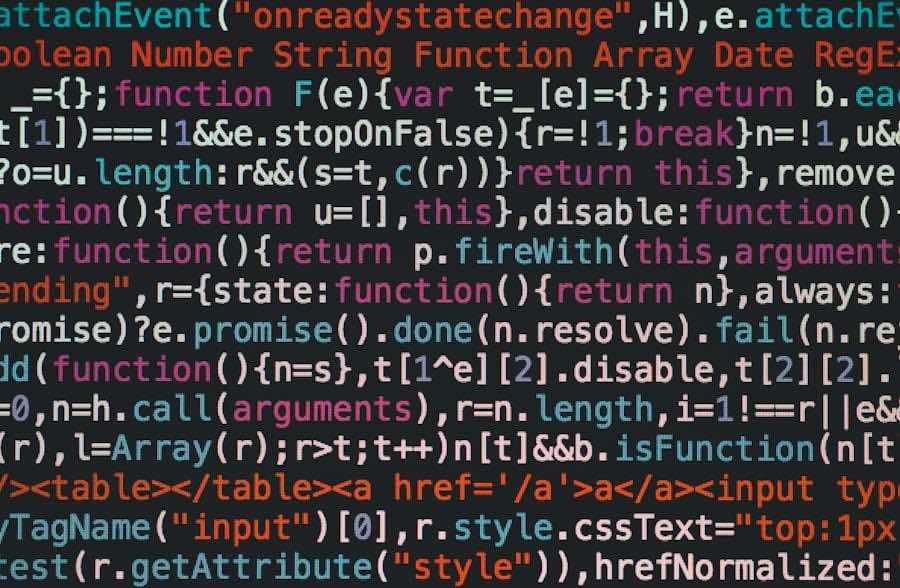
The real-world applications of quantum machine learning are beginning to emerge as researchers and companies explore its potential across various sectors. In finance, for instance, QML could revolutionise risk assessment and portfolio optimisation by enabling faster analysis of market data and more accurate predictions of asset behaviour. Financial institutions are already investing in QML research to gain a competitive edge in algorithmic trading and fraud detection.
In the realm of cybersecurity, QML has the potential to enhance encryption methods through advanced algorithms that can identify vulnerabilities more effectively than classical systems. As cyber threats become increasingly sophisticated, leveraging quantum computing’s capabilities could provide a significant advantage in safeguarding sensitive information. Furthermore, industries such as telecommunications and energy are also exploring QML applications for optimising network performance and improving resource allocation.
Future Developments in Quantum Machine Learning
As the field of quantum machine learning continues to evolve, several key developments are anticipated in the coming years. One area of focus will be the refinement of quantum hardware to improve qubit stability and reduce error rates. Advances in materials science and engineering may lead to more reliable quantum systems capable of supporting complex QML algorithms without significant loss of fidelity.
Additionally, as more researchers enter the field, we can expect an increase in collaborative efforts aimed at developing standardised frameworks for QML algorithms. This standardisation will facilitate knowledge sharing and accelerate progress by providing a common foundation upon which researchers can build their work. Furthermore, educational initiatives aimed at training professionals in both quantum computing and machine learning will be essential for fostering a skilled workforce capable of driving innovation in this area.
The integration of artificial intelligence with quantum computing is also likely to yield exciting developments. As AI systems become more sophisticated, their synergy with QML could lead to new paradigms in data analysis and decision-making processes across various industries.
Implications for the Future of Artificial Intelligence
The implications of quantum machine learning for the future of artificial intelligence are profound and far-reaching. As QML matures, it has the potential to enhance AI capabilities significantly by enabling faster processing times and more complex model training. This could lead to breakthroughs in areas such as natural language processing, computer vision, and autonomous systems, where traditional methods may struggle with large datasets or intricate patterns.
Moreover, the ability to analyse data at unprecedented speeds could facilitate real-time decision-making processes across various applications, from smart cities to autonomous vehicles. As AI systems become more adept at understanding complex data relationships through QML techniques, we may witness a new era of intelligent systems capable of adapting and evolving based on their environments. However, these advancements also raise ethical considerations regarding data privacy and security.
As AI systems become increasingly powerful through QML integration, ensuring responsible use and safeguarding against misuse will be paramount. The future landscape will require careful navigation as society grapples with the implications of these technologies on privacy, security, and ethical standards. In summary, while quantum machine learning is still in its infancy, its potential to reshape artificial intelligence is undeniable.
As research progresses and practical applications emerge, we stand on the brink of a new frontier in technology that could redefine our understanding of computation and intelligence itself.
For businesses looking to stay ahead of the curve, the article “5 Ways to Upgrade Your Office Space” offers valuable insights on creating a modern and efficient work environment. Just as Quantum Machine Learning is revolutionizing the field of artificial intelligence, upgrading office spaces can enhance productivity and employee satisfaction. By incorporating innovative design elements and technology, companies can create a workspace that fosters creativity and collaboration.
FAQs
What is quantum machine learning?
Quantum machine learning is the integration of quantum computing and artificial intelligence. It involves using quantum algorithms and quantum computing techniques to enhance machine learning processes.
How does quantum machine learning differ from classical machine learning?
Quantum machine learning differs from classical machine learning in that it leverages the principles of quantum mechanics to perform computations. This allows for the processing of large amounts of data and the solving of complex problems at a much faster rate than classical machine learning.
What are the potential benefits of quantum machine learning?
The potential benefits of quantum machine learning include the ability to process and analyse large datasets more efficiently, solve complex optimization problems, and improve the accuracy of machine learning models.
What are some applications of quantum machine learning?
Some potential applications of quantum machine learning include drug discovery, financial modelling, supply chain optimization, and the development of more advanced AI systems.
What are the current challenges in quantum machine learning?
Some of the current challenges in quantum machine learning include the need for more powerful and reliable quantum hardware, the development of quantum algorithms that can outperform classical algorithms, and the integration of quantum machine learning techniques into existing AI systems.
How is quantum machine learning being researched and developed?
Quantum machine learning is being researched and developed by a combination of academic institutions, research labs, and technology companies. These efforts involve exploring new quantum algorithms, developing quantum hardware, and investigating potential applications of quantum machine learning.