The advent of artificial intelligence (AI) has ushered in a new era in healthcare, particularly through the lens of clinical analytics. AI-powered clinical analytics refers to the application of advanced algorithms and machine learning techniques to analyse vast amounts of healthcare data, enabling healthcare professionals to derive actionable insights. This technology harnesses the power of data, transforming it into a resource that can significantly enhance decision-making processes, improve patient outcomes, and streamline operations within healthcare systems.
As the volume of health data continues to grow exponentially, the integration of AI into clinical analytics becomes increasingly vital for effective healthcare delivery. The significance of AI-powered clinical analytics lies not only in its ability to process large datasets but also in its potential to identify patterns and trends that may not be immediately apparent to human analysts. By leveraging historical patient data, treatment outcomes, and demographic information, AI can assist clinicians in making more informed decisions.
This capability is particularly crucial in an era where precision medicine is gaining traction, as it allows for tailored treatment plans that consider individual patient characteristics. The promise of AI in clinical analytics is not merely theoretical; numerous case studies and pilot programmes have demonstrated its efficacy in various healthcare settings, paving the way for broader adoption.
Summary
- AI-powered clinical analytics uses artificial intelligence to analyse clinical data and improve patient care in healthcare settings.
- Artificial intelligence plays a crucial role in healthcare by assisting in diagnosis, treatment planning, and patient monitoring.
- AI-powered clinical analytics improves patient care by providing personalised treatment plans, predicting potential health issues, and reducing medical errors.
- Implementing AI-powered clinical analytics can lead to cost savings in healthcare by streamlining processes, reducing unnecessary tests, and improving resource allocation.
- Ethical considerations in AI-powered clinical analytics include patient privacy, data security, and the potential for bias in algorithms.
The Role of Artificial Intelligence in Healthcare
Artificial intelligence plays a multifaceted role in healthcare, encompassing a range of applications from diagnostics to operational efficiency. One of the most prominent uses of AI is in diagnostic imaging, where machine learning algorithms can analyse radiological images with remarkable accuracy. For instance, AI systems have been developed to detect anomalies in X-rays and MRIs, often achieving performance levels comparable to or exceeding those of experienced radiologists.
This capability not only enhances diagnostic accuracy but also reduces the time required for image interpretation, allowing clinicians to focus on patient care rather than administrative tasks. Beyond diagnostics, AI is also instrumental in predictive analytics, which involves forecasting patient outcomes based on historical data. By analysing patterns in patient records, AI can identify individuals at high risk for conditions such as diabetes or heart disease, enabling early intervention strategies.
For example, a study published in the journal “Nature” demonstrated that machine learning models could predict hospital readmissions with a high degree of accuracy by analysing factors such as previous admissions, comorbidities, and social determinants of health. This predictive capability empowers healthcare providers to implement proactive measures, ultimately improving patient outcomes and reducing the burden on healthcare systems.
How AI-Powered Clinical Analytics Improves Patient Care
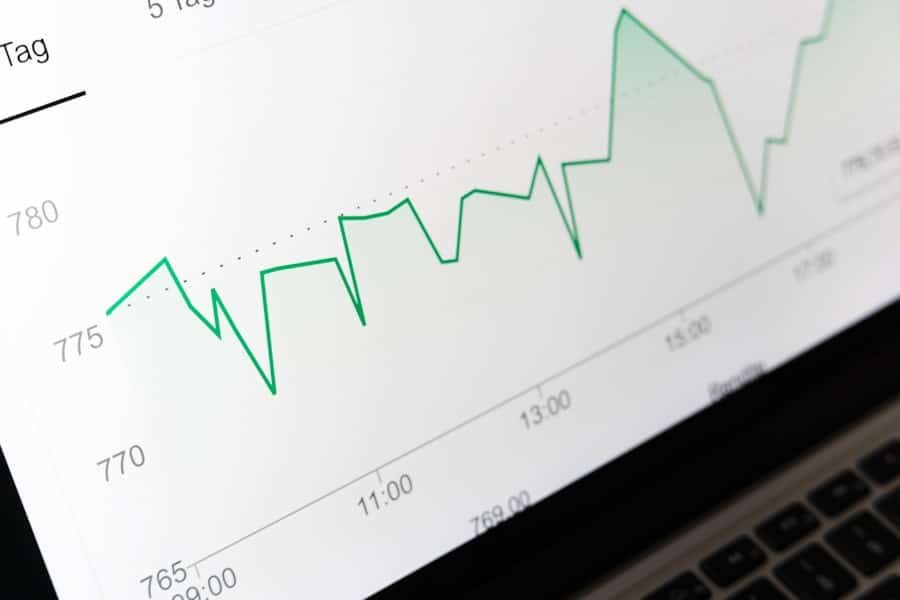
AI-powered clinical analytics enhances patient care through several mechanisms, primarily by facilitating personalised treatment plans and improving clinical decision-making. By analysing comprehensive datasets that include genetic information, lifestyle factors, and treatment histories, AI can help clinicians tailor interventions to meet the unique needs of each patient. For instance, in oncology, AI algorithms can analyse tumour genomics alongside patient demographics to recommend targeted therapies that are more likely to be effective for specific cancer types.
This level of precision not only increases the likelihood of successful treatment but also minimises unnecessary side effects associated with less targeted approaches. Moreover, AI-powered clinical analytics can significantly improve the efficiency of care delivery. By automating routine tasks such as data entry and appointment scheduling, healthcare providers can allocate more time to direct patient interactions.
For example, natural language processing (NLP) technologies can transcribe physician-patient conversations in real-time, allowing clinicians to focus on patient engagement rather than documentation. Additionally, AI can assist in monitoring patients remotely through wearable devices that track vital signs and other health metrics. This continuous monitoring enables timely interventions when abnormalities are detected, thereby enhancing overall patient safety and satisfaction.
The Impact of AI-Powered Clinical Analytics on Healthcare Costs
The integration of AI-powered clinical analytics into healthcare systems has the potential to significantly reduce costs while maintaining or even improving the quality of care. One of the primary ways this technology achieves cost savings is through enhanced operational efficiency. By streamlining administrative processes and optimising resource allocation, healthcare organisations can reduce overhead expenses.
For instance, predictive analytics can help hospitals manage bed occupancy rates more effectively by forecasting patient admissions based on historical trends, thus minimising unnecessary costs associated with overstaffing or underutilisation of resources. Furthermore, AI-powered clinical analytics can lead to substantial savings by reducing the incidence of adverse events and hospital readmissions. By identifying high-risk patients and implementing targeted interventions, healthcare providers can prevent complications that often result in costly emergency care or extended hospital stays.
A study conducted by the American Journal of Managed Care found that hospitals employing predictive analytics experienced a 20% reduction in readmission rates for heart failure patients. Such reductions not only alleviate financial strain on healthcare systems but also enhance patient experiences by minimising disruptions to their lives.
Ethical Considerations in AI-Powered Clinical Analytics
As with any transformative technology, the implementation of AI-powered clinical analytics raises important ethical considerations that must be addressed to ensure responsible use. One significant concern is data privacy and security. The vast amounts of sensitive health information processed by AI systems necessitate robust safeguards to protect patient confidentiality.
Healthcare organisations must adhere to stringent regulations such as the General Data Protection Regulation (GDPR) in Europe and the Health Insurance Portability and Accountability Act (HIPAA) in the United States to ensure that patient data is handled ethically and securely. Another ethical consideration revolves around algorithmic bias. If AI systems are trained on datasets that lack diversity or are skewed towards certain populations, there is a risk that these algorithms may produce biased outcomes that adversely affect underrepresented groups.
For instance, a study published in “Science” highlighted how facial recognition algorithms exhibited higher error rates for individuals with darker skin tones due to insufficient representation in training datasets. In healthcare, such biases could lead to disparities in treatment recommendations or diagnostic accuracy. Therefore, it is imperative for developers and healthcare providers to actively work towards creating inclusive datasets and continuously monitor AI systems for potential biases.
Challenges and Limitations of AI-Powered Clinical Analytics
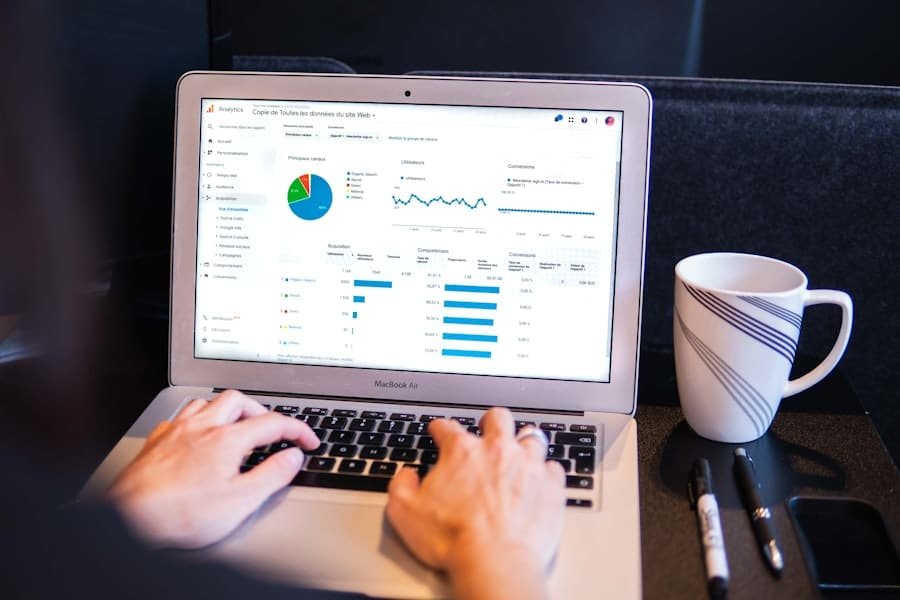
Despite its promising potential, the deployment of AI-powered clinical analytics is not without challenges and limitations. One major hurdle is the integration of disparate data sources within healthcare systems. Patient data often resides in various formats across multiple platforms—ranging from electronic health records (EHRs) to laboratory information systems—making it difficult for AI algorithms to access and analyse comprehensive datasets effectively.
This fragmentation can hinder the ability of AI systems to generate accurate insights and recommendations. Additionally, there exists a significant knowledge gap among healthcare professionals regarding the use of AI technologies. Many clinicians may lack familiarity with machine learning concepts or may be sceptical about relying on algorithms for clinical decision-making.
This apprehension can impede the adoption of AI-powered tools within clinical settings. To overcome this barrier, it is essential to provide adequate training and education for healthcare providers on how to interpret AI-generated insights and integrate them into their practice effectively.
Future Developments in AI-Powered Clinical Analytics
The future of AI-powered clinical analytics holds immense promise as advancements in technology continue to evolve at a rapid pace. One area poised for growth is the integration of real-time data analytics into clinical workflows. As wearable devices and remote monitoring technologies become increasingly prevalent, the ability to analyse real-time health data will enable clinicians to make immediate decisions based on current patient conditions rather than relying solely on historical information.
This shift could lead to more dynamic and responsive care models that adapt to patients’ needs as they change. Moreover, advancements in natural language processing are expected to enhance the capabilities of AI systems further. By improving the ability of algorithms to understand and interpret unstructured data—such as physician notes or patient feedback—AI can provide deeper insights into patient experiences and treatment efficacy.
This evolution will facilitate a more holistic approach to patient care that considers not only clinical metrics but also qualitative factors that contribute to overall well-being.
The Potential of AI-Powered Clinical Analytics in Transforming Healthcare
The transformative potential of AI-powered clinical analytics is evident across various facets of healthcare delivery. From enhancing diagnostic accuracy to optimising resource allocation and personalising treatment plans, the applications of this technology are vast and varied. As healthcare systems continue to grapple with increasing demands for efficiency and quality care, embracing AI-powered solutions will be crucial for meeting these challenges head-on.
However, it is essential for stakeholders—including healthcare providers, policymakers, and technology developers—to navigate the ethical considerations and challenges associated with implementing AI technologies responsibly. By fostering collaboration among these groups and prioritising transparency and inclusivity in data practices, the full potential of AI-powered clinical analytics can be realised, ultimately leading to improved patient outcomes and a more sustainable healthcare system for future generations.
AI-Powered Clinical Analytics is revolutionising the healthcare industry by providing valuable insights and improving patient outcomes. In a related article on competitive advantage here, businesses can learn how to leverage their strengths to stay ahead of the competition. Just like how AI is transforming clinical analytics, understanding and utilising competitive advantages can help companies thrive in their respective industries.
FAQs
What is AI-Powered Clinical Analytics?
AI-Powered Clinical Analytics refers to the use of artificial intelligence (AI) technology to analyse clinical data and provide insights that can improve patient care, streamline operations, and support decision-making in healthcare settings.
How does AI-Powered Clinical Analytics work?
AI-Powered Clinical Analytics works by using machine learning algorithms to analyse large volumes of clinical data, such as electronic health records, medical imaging, and genomic data. The AI algorithms can identify patterns, trends, and correlations in the data that may not be readily apparent to human analysts.
What are the benefits of AI-Powered Clinical Analytics?
The benefits of AI-Powered Clinical Analytics include improved accuracy and efficiency in diagnosing and treating patients, better prediction of patient outcomes, reduced healthcare costs, and enhanced operational efficiency in healthcare organisations.
What are some examples of AI-Powered Clinical Analytics applications?
Examples of AI-Powered Clinical Analytics applications include predictive analytics for identifying patients at risk of developing certain conditions, image recognition for diagnosing medical imaging scans, and natural language processing for extracting insights from unstructured clinical notes.
What are the challenges of implementing AI-Powered Clinical Analytics?
Challenges of implementing AI-Powered Clinical Analytics include concerns about data privacy and security, the need for robust validation and regulation of AI algorithms, and the requirement for healthcare professionals to be trained in interpreting and using AI-generated insights.