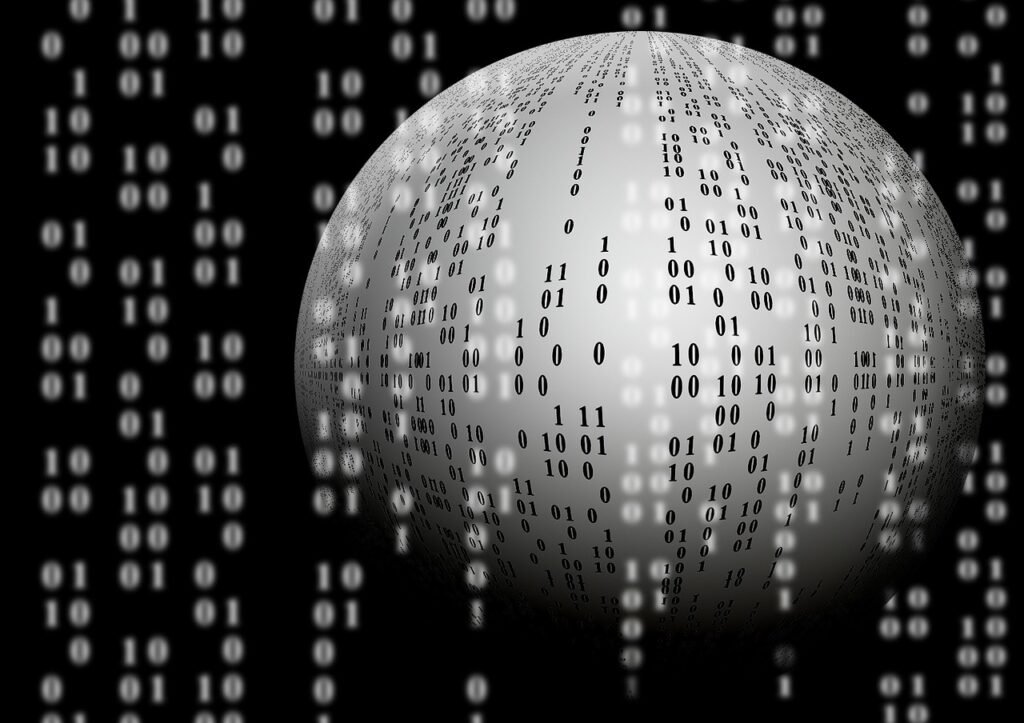
Master Data Management is essential in a world obsessed with data holding and storage. It is no longer a “thing to think about in the future” but rather an urgent, necessary process that all businesses must go through in order to make sense of their data. Data cleansing is highly recommended to get the hang of your master data without any hitch.
The way we learn, unlearn and relearn as humans is the same way organizations are meant to declutter data or unnecessary information from their master data. It helps keep the data efficiently and in good shape and quality.
Maintaining data quality is a serious task for organization data aggregators. They invest heavily in keeping contact lists, profiles, products, customers, sales, demographics, and other data clean, accurate, and up to date. According to HBR, 47% of newly created data records contain at least one significant error that interferes with work. And we’re talking about new data in databases here, not old documents that everyone knows need cleaning up and enrichment.
Validation of high-quality data gathered from various sources is required, making data cleansing a mandatory and ongoing operation for data aggregators.
Synopps cleansing can assist you in automating your data cleansing processes. As a business owner, you’ll likely be dealing with a variety of data sources for your enterprise. These sources can be grouped in silos. When this happens, data becomes more challenging to organize, which leads to errors and the side effects of data impurity discussed above.
This article will help you with tips for your organization’s master data cleansing. In no particular order are the steps you should take to cleanse your Master data.
1. Developing a data quality strategy
Mapping out a plan is essential for any project, including data cleansing. With 27% of data aggregators or stewards unsure about the accuracy of their database, it is needed to develop a data quality plan to establish a realistic and concrete baseline of data hygiene. The factors that can cause the strategy to differ include:
- Cleaning static databases at the backend before they enter the system.
- Cleaning real-time data streams.
- Their source.
- Their destination.
The way in which you can develop a data cleansing strategy to maintain the quality of your B2B database is; by Choosing and focusing on a model and metrics, developing key performance indicators (KPIs) for data quality to track data health, defining the initial data inspection procedure and quick sample checks are performed to identify complex datasets, Choosing the level of detail, tools, creating checks to prevent data over-cleaning, Begin data cleansing and error correction.
A plausibility analysis and comparison of new data to previous sets are used to assess the overall data quality. Errors can cause anomalies in patterns and outliers just as quickly as they can cause irregularities in patterns.
2. Verify your data
When you audit your master data on a regular basis, you are constantly looking for viruses or errors in the data. Without regular audits, it is impossible to identify solutions that are needed to help you out.
Data auditing can be time-consuming. Manually, it is a difficult task. Data cleansing tools should be used.
Auditing the data you use and require is a great idea. You may discover that some data sources clog your processes. There isn’t one! Auditing your data and carefully calculating its ROI can help. Around 27% of business leaders are
3. Keep track of errors
Keep track of the trends that lead to the majority of your errors.
This will make identifying and correcting incorrect or corrupt data much easier. Records are especially important if you’re integrating other solutions with your fleet management software to avoid clogging up the work of other departments.
4. Every database degrades
According to a Vainu study, 30% of company Master data becomes outdated each year. Many factors contribute to this, such as changing email addresses and home addresses, getting new phone numbers, leaving organizations, and changing job titles.
It’s best to keep your data updated by implementing some strategies. This can be done by employing parsing tools, which scan through all incoming emails and update contact information as it becomes available.
As a result, if another company hires a contact, your central database will be instantly updated. We would also advise deleting any email addresses that have bounced or opted out — this type of information is almost certainly available in your email marketing tool.
5. Standardize data entry
Data standards should be normalized and implemented for company-wide data entry. It would be best if you created rules that will tell in what way or forms values should be input into your master data and folders to put them. Ensure employees know how to check for a duplicated copy before creating a new contact, and also make them familiar with the app for entering data. This helps save time when entering data or searching for data.
By following these tactics, you can ensure you have a much cleaner and more organized contacts database. Also, syncing the data between your critical business applications helps minimize manual data entry and allows you to always get the most up-to-date and accurate contact information in all your tools.
6. Making use of imputation techniques to address missing values
One of the most challenging aspects of data cleansing is dealing with missing values, which distort analytic results. The simplest solution is to remove the columns that have missing values. When working with large datasets, list-wise and pair-wise deletions are ineffective. Regression is another method for dealing with missing data that requires a lot of computing power.
Migrate to machine-learning-based processes, and imputation techniques are examples of current data imputation techniques.
Before treating missing values, investigate and identify various imputation techniques.
Whenever possible, use average imputation and common point imputation.
By now, you should know that master data cleansing is necessary for an organization. And data cleansing helps keep your data updated and quality for use. There are ways to cleanse your data, and one of their most important ways is to always create rules and regulations that guide your master data.