In recent years, the financial services industry has witnessed a transformative shift with the integration of machine learning (ML) into risk management practices. This evolution is driven by the need for more sophisticated tools to navigate the complexities of modern financial landscapes, characterised by vast amounts of data and rapidly changing market conditions. Machine learning, a subset of artificial intelligence, enables systems to learn from data patterns and make predictions or decisions without explicit programming.
This capability is particularly valuable in risk management, where the ability to anticipate potential threats and mitigate them proactively can significantly enhance an organisation’s resilience and profitability. The application of machine learning in risk management encompasses various domains, including credit risk assessment, fraud detection, market risk management, operational risk management, and compliance. Each of these areas presents unique challenges that machine learning can address through advanced analytical techniques.
By leveraging algorithms that can process large datasets and identify intricate patterns, financial institutions can improve their risk assessment processes, enhance decision-making, and ultimately safeguard their assets against potential losses. As the financial sector continues to evolve, the role of machine learning in risk management is expected to expand, offering new opportunities for innovation and efficiency.
Summary
- Machine learning is revolutionizing risk management by providing advanced tools for predictive analytics and decision-making.
- In credit risk assessment, machine learning algorithms can analyse large volumes of data to accurately predict the creditworthiness of borrowers.
- Machine learning is highly effective in fraud detection and prevention by identifying patterns and anomalies in transaction data.
- Predictive analytics using machine learning can help in assessing market risk by forecasting potential fluctuations and identifying trends.
- Operational risk management can benefit from machine learning by automating processes and identifying potential risks in real-time.
Machine Learning Applications in Credit Risk Assessment
Credit risk assessment is a critical component of financial institutions’ operations, as it determines the likelihood that a borrower will default on a loan. Traditional credit scoring models often rely on historical data and simplistic statistical methods, which may not capture the full spectrum of factors influencing creditworthiness. Machine learning offers a more nuanced approach by utilising complex algorithms that can analyse diverse datasets, including transaction histories, social media activity, and even alternative data sources such as utility payments or rental history.
This allows for a more comprehensive evaluation of an individual’s credit risk profile. For instance, companies like ZestFinance have pioneered the use of machine learning in credit scoring by developing models that incorporate non-traditional data points. By analysing thousands of variables, these models can identify patterns that traditional scoring methods might overlook.
This not only enhances the accuracy of credit assessments but also expands access to credit for individuals who may have been previously deemed uncreditworthy due to insufficient credit history. Furthermore, machine learning models can be continuously updated with new data, allowing lenders to refine their assessments in real-time and respond swiftly to changing borrower circumstances.
Machine Learning for Fraud Detection and Prevention
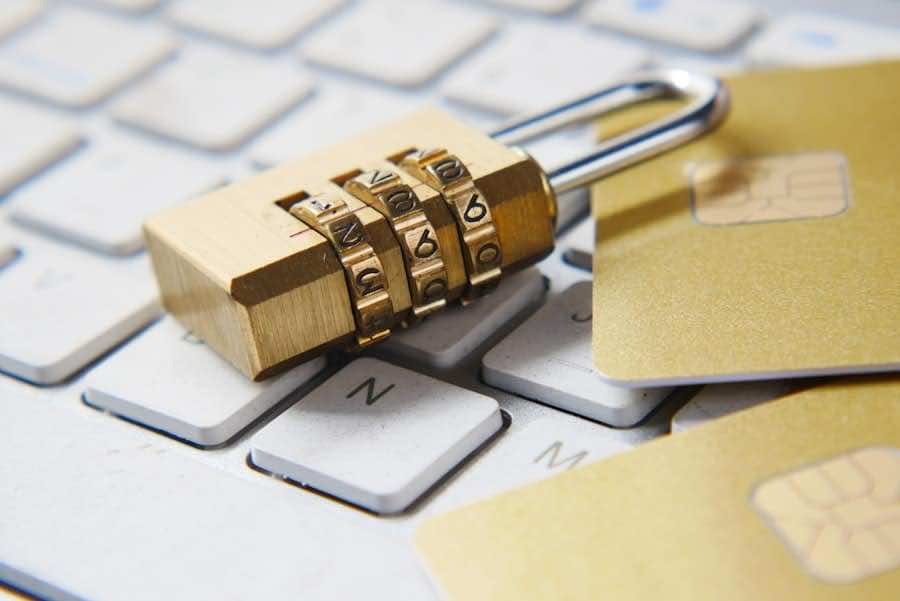
Fraud detection is another area where machine learning has made significant strides. Financial institutions face an ever-evolving landscape of fraudulent activities, from credit card fraud to identity theft. Traditional rule-based systems often struggle to keep pace with sophisticated fraud schemes, leading to either false positives or missed detections.
Machine learning algorithms excel in this domain by analysing transaction patterns and identifying anomalies that may indicate fraudulent behaviour. For example, PayPal employs machine learning techniques to monitor transactions in real-time. By training models on historical transaction data, the system learns to distinguish between legitimate and potentially fraudulent activities.
When a transaction deviates from established patterns such as an unusually large purchase from a new device or location the system flags it for further investigation. This proactive approach not only helps in reducing financial losses but also enhances customer trust by minimising false declines of legitimate transactions. Moreover, as these models learn from new data continuously, they become increasingly adept at recognising emerging fraud trends, thereby improving their effectiveness over time.
Predictive Analytics for Market Risk Management
Market risk management involves assessing the potential losses that could arise from fluctuations in market prices, interest rates, or foreign exchange rates. The complexity of financial markets necessitates advanced analytical tools capable of processing vast amounts of data and generating accurate forecasts. Machine learning provides powerful predictive analytics capabilities that can enhance market risk assessment by identifying trends and correlations that may not be immediately apparent through traditional analysis.
One notable application is in the realm of algorithmic trading, where firms utilise machine learning models to predict price movements based on historical data and real-time market indicators. For instance, hedge funds like Renaissance Technologies employ sophisticated algorithms that analyse millions of data points to identify trading opportunities with high probability outcomes. These models can adapt to changing market conditions by recalibrating their predictions based on new information, allowing traders to make informed decisions swiftly.
Additionally, machine learning can assist in stress testing scenarios by simulating various market conditions and assessing potential impacts on portfolios, thereby enabling firms to develop robust risk mitigation strategies.
Machine Learning for Operational Risk Management
Operational risk encompasses the potential losses resulting from inadequate or failed internal processes, systems, or external events. This type of risk is often challenging to quantify due to its diverse nature and the difficulty in predicting human behaviour or system failures. Machine learning offers innovative solutions for operational risk management by enabling organisations to analyse historical incident data and identify underlying patterns that contribute to operational failures.
For example, banks can utilise machine learning algorithms to analyse past operational incidents—such as system outages or compliance breaches—to identify common factors that led to these events. By recognising these patterns, organisations can implement targeted interventions to mitigate future risks. Additionally, predictive maintenance powered by machine learning can enhance operational resilience by forecasting equipment failures before they occur.
This proactive approach not only minimises downtime but also reduces costs associated with emergency repairs and lost productivity.
Application of Machine Learning in Compliance and Regulatory Risk
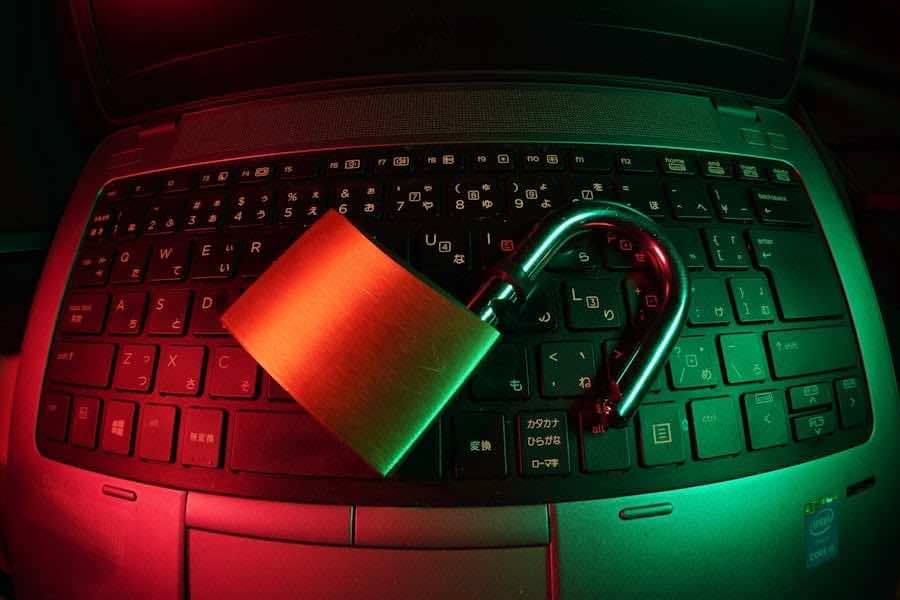
Compliance with regulatory requirements is a critical aspect of risk management for financial institutions. The increasing complexity of regulations necessitates robust systems capable of monitoring compliance effectively. Machine learning can streamline compliance processes by automating the analysis of vast amounts of regulatory data and identifying potential areas of non-compliance.
For instance, anti-money laundering (AML) efforts have greatly benefited from machine learning applications. Financial institutions are required to monitor transactions for suspicious activity continuously; however, manual reviews are often insufficient due to the sheer volume of transactions processed daily. Machine learning algorithms can analyse transaction patterns and flag anomalies that may indicate money laundering activities.
By automating this process, institutions can enhance their compliance efforts while reducing the burden on human resources. Furthermore, these models can adapt to evolving regulatory landscapes by incorporating new rules and guidelines into their analyses.
Challenges and Limitations of Machine Learning in Risk Management
Despite its numerous advantages, the integration of machine learning into risk management is not without challenges. One significant concern is the issue of data quality and availability. Machine learning models rely heavily on high-quality data for training; however, financial institutions often grapple with incomplete or inconsistent datasets.
Poor data quality can lead to inaccurate predictions and undermine the effectiveness of risk management strategies. Another challenge lies in the interpretability of machine learning models. Many advanced algorithms function as “black boxes,” making it difficult for practitioners to understand how decisions are made.
This lack of transparency can pose regulatory challenges, particularly in industries where accountability is paramount. Financial institutions must strike a balance between leveraging complex algorithms for improved accuracy while ensuring that their decision-making processes remain transparent and justifiable.
Future Trends and Opportunities in Machine Learning for Risk Management
Looking ahead, the future of machine learning in risk management appears promising as technological advancements continue to unfold. One emerging trend is the increased use of explainable AI (XAI), which aims to enhance the interpretability of machine learning models without sacrificing performance. By developing models that provide insights into their decision-making processes, financial institutions can improve transparency and build trust with regulators and stakeholders.
Additionally, the integration of machine learning with other technologies such as blockchain could revolutionise risk management practices further. Blockchain’s immutable ledger could provide a secure foundation for data sharing among institutions while machine learning algorithms analyse this data for insights into risk exposure and compliance adherence. Moreover, as organisations increasingly adopt cloud computing solutions, they will gain access to scalable resources that facilitate more extensive data analyses and model training.
This shift will enable financial institutions to harness real-time data streams more effectively, enhancing their ability to respond swiftly to emerging risks. In conclusion, as machine learning continues to evolve within the realm of risk management, it presents an array of opportunities for financial institutions seeking to enhance their resilience against potential threats while navigating an increasingly complex regulatory landscape. The ongoing development of innovative algorithms and technologies will undoubtedly shape the future of risk management practices across the industry.
Machine learning applications in risk management are becoming increasingly important for businesses in today’s fast-paced world. As discussed in a recent article on how technology is allowing businesses to keep delivering during this crisis, the use of advanced algorithms and predictive analytics can help companies identify and mitigate potential risks before they escalate. By leveraging machine learning tools, organisations can make more informed decisions and better protect their assets. This is just one example of how technology is revolutionising the way businesses operate and manage risk.
FAQs
What is machine learning?
Machine learning is a type of artificial intelligence that enables systems to learn and improve from experience without being explicitly programmed. It involves the use of algorithms to parse data, learn from it, and then make predictions or decisions based on that data.
What is risk management?
Risk management is the process of identifying, assessing, and prioritising potential risks or uncertainties in a business or investment decision. It involves taking steps to mitigate or manage these risks to minimise potential negative impacts.
How is machine learning used in risk management?
Machine learning is used in risk management to analyse large volumes of data and identify patterns or trends that may indicate potential risks. It can be used to predict credit defaults, detect fraudulent activities, assess market risks, and make investment decisions.
What are some machine learning applications in risk management?
Some machine learning applications in risk management include credit scoring, fraud detection, underwriting, portfolio management, and regulatory compliance. Machine learning algorithms can help automate and improve these processes by analysing data and making predictions.
What are the benefits of using machine learning in risk management?
The benefits of using machine learning in risk management include improved accuracy in risk assessment, faster decision-making processes, reduced human error, and the ability to handle large volumes of data. It can also help identify new types of risks that may not be apparent through traditional methods.
Are there any challenges in using machine learning for risk management?
Some challenges in using machine learning for risk management include the need for high-quality data, the potential for algorithmic biases, and the complexity of interpreting machine learning models. Additionally, regulatory and ethical considerations must be taken into account when using machine learning in risk management.