In the contemporary landscape of data-driven decision-making, AI-driven sentiment forecasting has emerged as a pivotal tool for businesses seeking to navigate the complexities of consumer emotions and opinions. This innovative approach leverages advanced algorithms and machine learning techniques to analyse vast amounts of textual data, enabling organisations to gauge public sentiment with remarkable accuracy. As the digital age continues to proliferate, the ability to understand and predict consumer sentiment has become not just advantageous but essential for maintaining competitive advantage.
The integration of artificial intelligence into sentiment analysis allows for a more nuanced understanding of consumer behaviour, providing insights that can inform marketing strategies, product development, and customer engagement. The significance of sentiment forecasting extends beyond mere data analysis; it encapsulates the essence of human emotion and its impact on market dynamics. By harnessing AI technologies, businesses can sift through social media posts, customer reviews, and other forms of user-generated content to extract valuable insights about public perception.
This capability is particularly crucial in an era where consumer opinions can shift rapidly, influenced by social trends, economic factors, and global events. As such, AI-driven sentiment forecasting not only aids in understanding current sentiments but also plays a vital role in predicting future trends, allowing companies to adapt proactively rather than reactively.
Summary
- AI-driven sentiment forecasting uses artificial intelligence to predict and analyse public sentiment towards a particular topic or brand.
- Artificial intelligence plays a crucial role in sentiment analysis by processing large volumes of data from various sources to understand and interpret human emotions and opinions.
- Sentiment forecasting is important for businesses as it helps them understand customer satisfaction, brand perception, and market trends, allowing for better decision-making and strategy development.
- AI-driven sentiment forecasting works by using natural language processing and machine learning algorithms to analyse text data from social media, customer reviews, and other sources to determine sentiment.
- AI-driven sentiment forecasting has applications in various industries such as marketing, finance, customer service, and healthcare, providing valuable insights for improving products and services.
The Role of Artificial Intelligence in Sentiment Analysis
Artificial intelligence serves as the backbone of modern sentiment analysis, employing sophisticated algorithms that can process and interpret human language with increasing precision. Traditional methods of sentiment analysis often relied on basic keyword matching or manual coding, which were limited in their ability to capture the subtleties of language, such as sarcasm or context-dependent meanings. In contrast, AI-driven approaches utilise natural language processing (NLP) techniques that enable machines to understand and analyse text in a manner akin to human comprehension.
This advancement allows for a more comprehensive evaluation of sentiments expressed in various forms of communication. Machine learning models, particularly those based on deep learning architectures, have revolutionised sentiment analysis by enabling systems to learn from vast datasets. These models can identify patterns and correlations within the data that may not be immediately apparent to human analysts.
For instance, a neural network trained on thousands of product reviews can discern not only the overall sentiment but also the specific aspects of a product that consumers feel positively or negatively about. This level of granularity is invaluable for businesses aiming to refine their offerings based on consumer feedback. Furthermore, AI can continuously improve its accuracy over time by learning from new data inputs, making it an ever-evolving tool in the realm of sentiment analysis.
Understanding the Importance of Sentiment Forecasting in Business
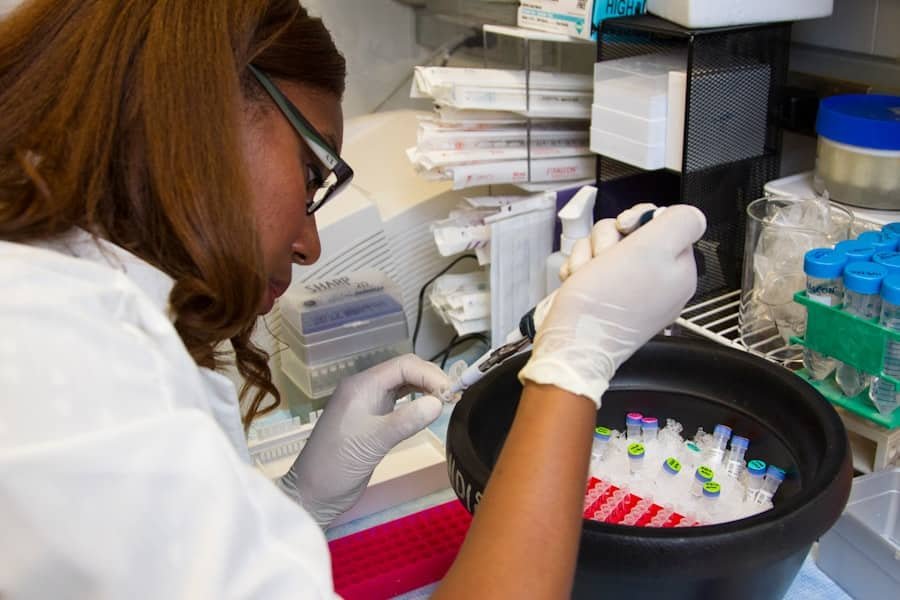
Sentiment forecasting plays a crucial role in shaping business strategies across various sectors. By accurately predicting consumer sentiment, organisations can make informed decisions that align with market demands and consumer expectations. For instance, a company launching a new product can utilise sentiment forecasting to gauge potential reactions from target audiences before the official release.
This proactive approach allows businesses to tailor their marketing campaigns and product features based on anticipated consumer responses, thereby minimising the risk of failure. Moreover, sentiment forecasting can significantly enhance customer relationship management. By understanding how customers feel about their experiences with a brand, companies can identify areas for improvement and address concerns before they escalate into larger issues.
For example, if sentiment analysis reveals a growing dissatisfaction with customer service interactions, a business can implement training programmes or process changes to enhance the customer experience. This responsiveness not only fosters customer loyalty but also cultivates a positive brand image in an increasingly competitive marketplace.
How AI-Driven Sentiment Forecasting Works
The mechanics of AI-driven sentiment forecasting involve several key processes that transform raw data into actionable insights. Initially, data is collected from various sources such as social media platforms, online reviews, blogs, and forums. This unstructured data is then pre-processed to remove noise and irrelevant information, ensuring that the subsequent analysis focuses on meaningful content.
Techniques such as tokenisation, stemming, and lemmatisation are employed during this stage to prepare the text for analysis. Once the data is cleaned and structured, machine learning algorithms are applied to classify sentiments as positive, negative, or neutral. This classification process often involves training models on labelled datasets where human annotators have already identified sentiments.
The trained model learns to recognise patterns associated with different sentiments and can then apply this knowledge to new, unseen data. Advanced techniques such as sentiment scoring and aspect-based sentiment analysis further enhance the depth of insights generated by these models. For instance, aspect-based analysis allows businesses to understand not just whether sentiments are positive or negative but also which specific features or attributes are driving those sentiments.
Applications of AI-Driven Sentiment Forecasting in Different Industries
AI-driven sentiment forecasting finds applications across a multitude of industries, each leveraging its capabilities to enhance decision-making processes. In the retail sector, for example, companies utilise sentiment analysis to monitor customer feedback on products and services in real-time. By analysing social media conversations and online reviews, retailers can quickly identify trends in consumer preferences and adjust their inventory or marketing strategies accordingly.
A notable example is how fashion retailers track social media influencers’ sentiments towards their brands to inform seasonal collections and promotional campaigns. In the financial services industry, sentiment forecasting plays a critical role in market analysis and investment strategies. Financial analysts employ sentiment analysis tools to assess public sentiment regarding stocks or economic indicators based on news articles and social media discussions.
For instance, during significant market events such as earnings reports or economic announcements, AI-driven sentiment analysis can provide insights into investor sentiment that may influence stock prices. This capability allows traders to make more informed decisions based on anticipated market movements rather than relying solely on historical data.
Benefits and Challenges of AI-Driven Sentiment Forecasting

The benefits of AI-driven sentiment forecasting are manifold, offering organisations enhanced capabilities in understanding consumer behaviour and market dynamics. One significant advantage is the speed at which insights can be generated; traditional methods of sentiment analysis often require extensive manual effort and time. In contrast, AI systems can process vast amounts of data within minutes or hours, providing businesses with timely information that can inform strategic decisions.
Additionally, the scalability of AI solutions means that organisations can analyse data from multiple sources simultaneously without compromising accuracy. However, despite its advantages, AI-driven sentiment forecasting is not without challenges. One major concern is the quality of the data being analysed; if the input data is biased or unrepresentative of the broader population, the resulting insights may lead to misguided conclusions.
Furthermore, while AI models have made significant strides in understanding language nuances, they still struggle with context-specific meanings and cultural references that may affect sentiment interpretation. This limitation underscores the importance of human oversight in the analysis process to ensure that insights are contextualised appropriately.
Ethical Considerations in AI-Driven Sentiment Forecasting
As with any technology that processes personal data and opinions, ethical considerations surrounding AI-driven sentiment forecasting are paramount. One primary concern is privacy; organisations must navigate the delicate balance between leveraging consumer data for insights while respecting individual privacy rights. The collection and analysis of user-generated content raise questions about consent and transparency; consumers should be informed about how their data is being used and have the option to opt out if they choose.
Moreover, there is a risk of reinforcing biases present in training datasets when developing AI models for sentiment analysis. If these models are trained predominantly on data from specific demographics or regions, they may inadvertently perpetuate stereotypes or overlook sentiments expressed by underrepresented groups. To mitigate these risks, organisations must adopt ethical frameworks that prioritise fairness and inclusivity in their AI practices.
This includes diversifying training datasets and implementing regular audits to assess model performance across different demographic groups.
Future Trends in AI-Driven Sentiment Forecasting
Looking ahead, several trends are poised to shape the future of AI-driven sentiment forecasting. One notable trend is the increasing integration of multimodal data sources into sentiment analysis frameworks. As consumers engage with brands across various platforms—textual content on social media, video reviews on YouTube, and audio feedback through podcasts—AI systems will need to evolve to analyse sentiments across these diverse formats effectively.
This shift will require advancements in natural language processing as well as image and audio recognition technologies. Another emerging trend is the growing emphasis on real-time sentiment analysis capabilities. As businesses strive for agility in responding to market changes and consumer feedback, there will be a heightened demand for tools that provide instantaneous insights into public sentiment.
This demand will likely drive innovations in AI algorithms that can process streaming data from social media platforms or news outlets as events unfold. Furthermore, as organisations become more aware of ethical considerations surrounding AI usage, there will be an increased focus on developing transparent and explainable AI models for sentiment forecasting. Stakeholders will seek clarity on how decisions are made based on AI-generated insights, fostering trust between consumers and brands while ensuring compliance with regulatory standards.
In conclusion, AI-driven sentiment forecasting represents a transformative force in how businesses understand and respond to consumer emotions and opinions. As technology continues to advance, its applications will expand across industries while addressing ethical considerations that arise from its use.
AI-Driven Sentiment Forecasting is a cutting-edge technology that is revolutionizing the way businesses understand and predict consumer behaviour. In a related article on analysing the impact of temperature on workplace productivity, researchers delve into the effects of environmental factors on employee performance. Just as AI can analyse sentiment to forecast consumer behaviour, understanding how temperature affects productivity can help businesses create a more efficient work environment. By leveraging both AI-driven sentiment forecasting and insights on workplace productivity, companies can gain a competitive edge in today’s fast-paced market.
FAQs
What is AI-Driven Sentiment Forecasting?
AI-Driven Sentiment Forecasting is a process of using artificial intelligence and machine learning algorithms to analyse and predict the sentiment of a particular group of people towards a specific topic, product, or service.
How does AI-Driven Sentiment Forecasting work?
AI-Driven Sentiment Forecasting works by collecting and analysing large amounts of data from various sources such as social media, customer reviews, and surveys. The AI algorithms then process this data to identify patterns and trends in sentiment, and make predictions about future sentiment towards the topic.
What are the benefits of AI-Driven Sentiment Forecasting?
Some of the benefits of AI-Driven Sentiment Forecasting include the ability to make more informed business decisions, understand customer preferences and opinions, and anticipate potential issues or opportunities.
What are some common applications of AI-Driven Sentiment Forecasting?
AI-Driven Sentiment Forecasting is commonly used in marketing and advertising to understand consumer attitudes towards products and brands. It is also used in customer service to identify and address customer concerns, and in financial markets to predict investor sentiment.
What are the limitations of AI-Driven Sentiment Forecasting?
Limitations of AI-Driven Sentiment Forecasting include the potential for bias in the data and algorithms, the inability to capture complex human emotions accurately, and the need for ongoing refinement and validation of the forecasting models.