The advent of smart grid technology has revolutionised the way energy is generated, distributed, and consumed. At the heart of this transformation lies smart grid analytics, a sophisticated approach that leverages data to enhance the efficiency, reliability, and sustainability of energy systems. Smart grid analytics encompasses a range of tools and techniques designed to analyse vast amounts of data generated by smart meters, sensors, and other devices integrated into the energy infrastructure.
This analytical capability enables utilities and energy providers to make informed decisions, optimise operations, and ultimately deliver better services to consumers. As the global demand for energy continues to rise, coupled with the pressing need for sustainable practices, the role of smart grid analytics becomes increasingly critical. By harnessing advanced analytics, energy providers can gain insights into consumption patterns, predict demand fluctuations, and identify potential outages before they occur.
This proactive approach not only enhances operational efficiency but also contributes to a more resilient energy grid capable of accommodating renewable energy sources and electric vehicles. The integration of smart grid analytics into the energy sector represents a significant leap towards a more intelligent and responsive energy ecosystem.
Summary
- Smart grid analytics involves the use of advanced technologies and data analysis to improve the efficiency and reliability of energy distribution.
- The benefits of smart grid analytics include improved grid reliability, better integration of renewable energy sources, and more efficient energy management.
- Data plays a crucial role in smart grid analytics, providing insights into energy consumption patterns, equipment performance, and potential issues in the grid.
- Implementing smart grid analytics in the energy sector requires investment in technology, data infrastructure, and skilled personnel.
- While there are challenges in implementing smart grid analytics, such as data security and privacy concerns, there are also opportunities for innovation and improved energy management in the future.
Understanding the Benefits of Smart Grid Analytics
The benefits of smart grid analytics are manifold, impacting various facets of the energy sector. One of the most significant advantages is the enhancement of operational efficiency. By analysing real-time data from smart meters and sensors, utilities can optimise their energy distribution networks, reducing waste and minimising losses.
For instance, predictive maintenance can be employed to identify equipment that is likely to fail, allowing for timely interventions that prevent costly outages and service disruptions. This not only saves money for utilities but also ensures a more reliable supply for consumers. Moreover, smart grid analytics facilitates better demand forecasting.
Utilities can analyse historical consumption data alongside external factors such as weather patterns and economic indicators to predict future energy needs with greater accuracy. This capability is particularly crucial in managing peak demand periods, where the risk of overload on the grid is heightened. By anticipating these spikes in demand, utilities can implement strategies such as demand response programmes, which incentivise consumers to reduce their usage during peak times.
This not only alleviates pressure on the grid but also promotes a more sustainable approach to energy consumption.
The Role of Data in Smart Grid Analytics
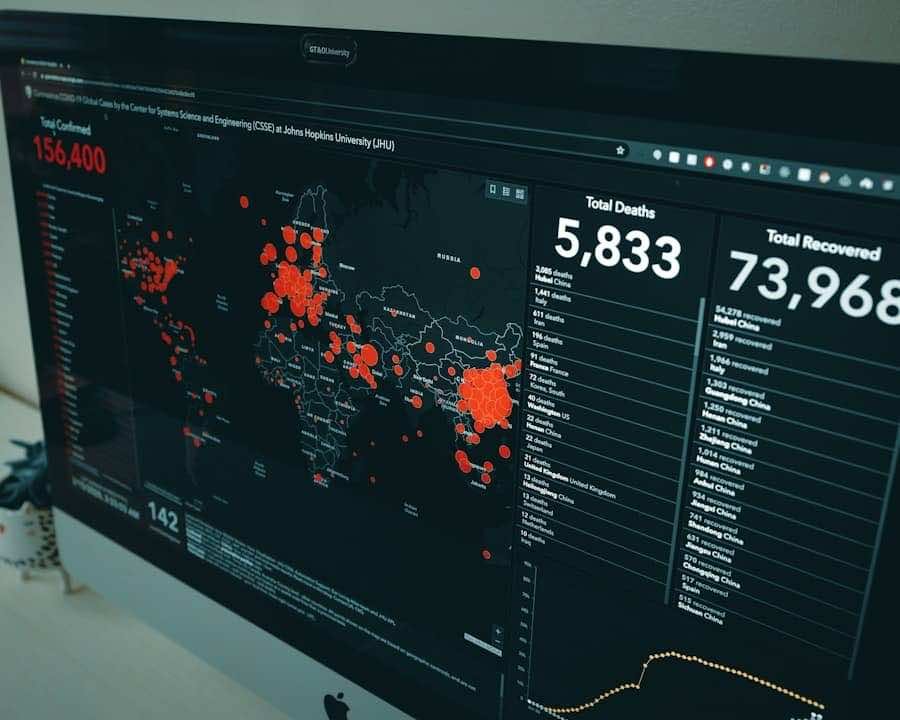
Data serves as the foundation upon which smart grid analytics operates. The proliferation of smart meters and IoT devices has resulted in an unprecedented volume of data being generated within the energy sector. This data encompasses a wide array of information, including real-time consumption patterns, voltage levels, and equipment performance metrics.
The challenge lies not only in collecting this data but also in effectively analysing it to extract actionable insights. Advanced analytics techniques, such as machine learning and artificial intelligence, play a pivotal role in processing this vast amount of information. These technologies enable utilities to identify trends and anomalies that may not be immediately apparent through traditional analysis methods.
For example, machine learning algorithms can be trained to recognise patterns in energy consumption that correlate with specific events or conditions, allowing for more accurate predictions and tailored responses. Furthermore, the integration of data from various sources such as weather forecasts and social media sentiment can enhance the contextual understanding of energy usage patterns, leading to more informed decision-making.
Implementing Smart Grid Analytics in the Energy Sector
The implementation of smart grid analytics within the energy sector requires a strategic approach that encompasses technology adoption, workforce training, and stakeholder engagement. Utilities must invest in robust data management systems capable of handling the influx of information generated by smart devices. This often involves upgrading existing infrastructure and integrating new technologies that facilitate real-time data collection and analysis.
Training personnel to effectively utilise these advanced analytical tools is equally important. A skilled workforce is essential for interpreting data insights and translating them into actionable strategies. Utilities may need to collaborate with technology providers or academic institutions to develop training programmes that equip employees with the necessary skills in data science and analytics.
Additionally, engaging with stakeholders including consumers, regulators, and policymakers—is crucial for fostering a culture of innovation and collaboration within the industry.
Challenges and Opportunities in Smart Grid Analytics
While the potential benefits of smart grid analytics are substantial, several challenges must be addressed to fully realise its advantages. One significant hurdle is data privacy and security. As utilities collect vast amounts of consumer data, concerns regarding how this information is stored and used become paramount.
Ensuring robust cybersecurity measures are in place is essential to protect sensitive information from breaches that could undermine consumer trust. Another challenge lies in the integration of disparate data sources. The energy sector comprises various stakeholders, each generating unique datasets that may not be easily compatible with one another.
Developing standardised protocols for data sharing and interoperability is crucial for creating a cohesive analytical framework that can leverage insights from multiple sources. However, these challenges also present opportunities for innovation. The demand for secure data management solutions has spurred advancements in cybersecurity technologies, while collaborative initiatives among industry players can lead to the development of best practices for data integration.
The Future of Smart Grid Analytics
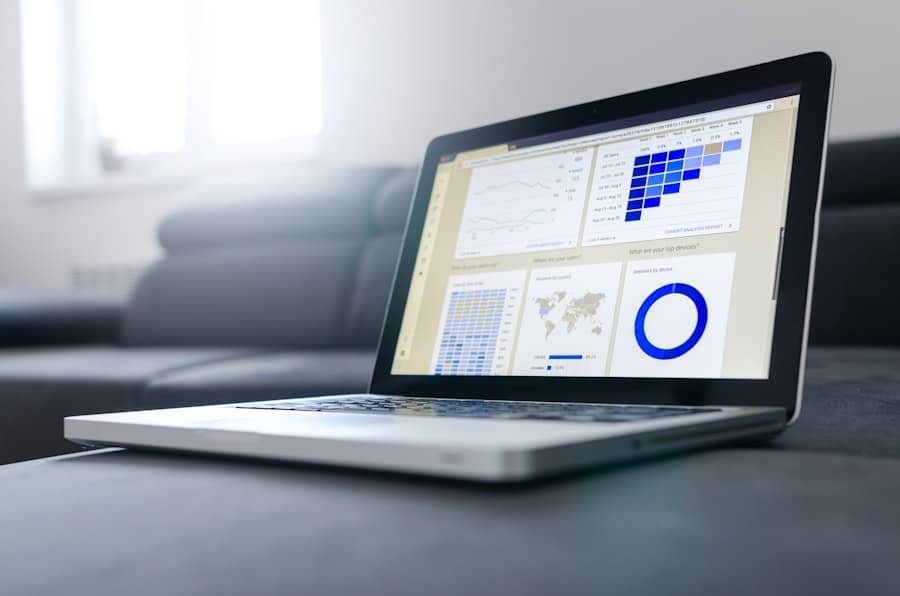
Looking ahead, the future of smart grid analytics appears promising as technological advancements continue to reshape the landscape of the energy sector. The increasing adoption of artificial intelligence and machine learning will further enhance predictive capabilities, enabling utilities to anticipate changes in demand with even greater precision. As these technologies evolve, they will likely become more accessible to smaller utilities that may have previously lacked the resources to implement sophisticated analytics solutions.
Moreover, the integration of renewable energy sources into the grid will necessitate more advanced analytical tools to manage variability and ensure stability. Smart grid analytics will play a crucial role in facilitating this transition by providing insights into how renewable generation interacts with traditional power sources. Additionally, as electric vehicles become more prevalent, analytics will be essential for managing charging infrastructure and optimising energy distribution during peak usage times.
Case Studies of Successful Smart Grid Analytics Implementation
Several case studies exemplify the successful implementation of smart grid analytics within the energy sector. One notable example is Pacific Gas and Electric (PG&E) in California, which has leveraged advanced analytics to enhance its wildfire prevention efforts. By analysing historical weather data alongside real-time sensor information from its power lines, PG&E has developed predictive models that identify high-risk areas for wildfires during extreme weather conditions.
This proactive approach has enabled the utility to implement targeted safety measures, such as de-energising power lines in high-risk zones during critical periods. Another compelling case is that of Enel Group in Italy, which has embraced smart grid analytics to optimise its operations across multiple countries. By utilising advanced data analytics platforms, Enel has been able to monitor its distribution network in real-time, identifying inefficiencies and potential outages before they escalate into significant issues.
This has resulted in improved service reliability and customer satisfaction while also reducing operational costs.
The Importance of Smart Grid Analytics in the Energy Industry
Smart grid analytics stands at the forefront of transforming the energy industry into a more efficient, reliable, and sustainable ecosystem. By harnessing vast amounts of data generated by modern technologies, utilities can optimise their operations, enhance customer engagement, and contribute to a greener future. As challenges such as data security and integration persist, they also pave the way for innovation and collaboration within the sector.
The future holds immense potential for smart grid analytics as it continues to evolve alongside advancements in technology and changing consumer behaviours. With successful case studies demonstrating its effectiveness, it is clear that embracing smart grid analytics is not merely an option but a necessity for utilities aiming to thrive in an increasingly complex energy landscape. The importance of this analytical approach cannot be overstated; it is integral to building a resilient energy infrastructure capable of meeting the demands of tomorrow while fostering sustainability today.
Smart Grid Analytics is a crucial tool for energy companies looking to optimise their operations and improve efficiency. According to a recent article on businesscasestudies.co.uk, the use of smart grid analytics can help companies reduce costs, increase reliability, and better manage their resources. By analysing data from smart meters, sensors, and other devices, energy companies can gain valuable insights into their operations and make more informed decisions. This article highlights the importance of smart grid analytics in the energy sector and how it can drive innovation and improve sustainability.
FAQs
What is Smart Grid Analytics?
Smart Grid Analytics is the use of advanced data analysis and predictive modelling techniques to extract valuable insights from the vast amount of data generated by smart grid systems. It helps utilities and energy companies to improve grid reliability, optimize energy distribution, and enhance overall operational efficiency.
How does Smart Grid Analytics work?
Smart Grid Analytics works by collecting and analyzing data from various sources within the smart grid, such as smart meters, sensors, and other grid devices. The data is then processed using advanced analytics tools to identify patterns, trends, and anomalies that can be used to make informed decisions and improve grid performance.
What are the benefits of Smart Grid Analytics?
Some of the benefits of Smart Grid Analytics include improved grid reliability, reduced energy losses, better demand forecasting, enhanced asset management, and more efficient energy distribution. It also enables utilities to better integrate renewable energy sources and support the transition to a more sustainable energy system.
What are some examples of Smart Grid Analytics applications?
Some examples of Smart Grid Analytics applications include predictive maintenance of grid infrastructure, real-time monitoring of grid performance, demand response management, outage prediction and management, and customer energy usage analysis. These applications help utilities to proactively address issues and optimize grid operations.
What are the challenges of implementing Smart Grid Analytics?
Challenges of implementing Smart Grid Analytics include managing and integrating large volumes of data from diverse sources, ensuring data security and privacy, developing and maintaining the necessary analytics capabilities, and addressing regulatory and compliance requirements. Additionally, there may be challenges related to the interoperability of different grid systems and devices.